Fake News Detection Using Machine Learning
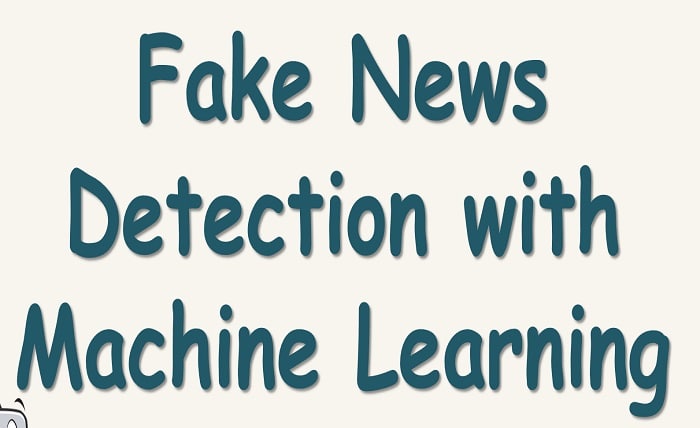
In today’s digital age, the spread of misinformation and fake news has become a significant challenge. The advent of social media platforms and online news portals has made it easier for false information to circulate rapidly, leading to potential consequences on individuals and society as a whole. However, with advancements in technology, particularly in the field of machine learning, we now have powerful tools at our disposal to combat this issue. In this article, we will explore the concept of fake news detection using machine learning and how it can help us distinguish between accurate information and misleading content.
The Fake News
What is Fake News?
Fake news refers to intentionally fabricated or misleading information that is presented as factual news. It can be created and spread through various mediums, including social media platforms, websites, and even traditional media channels. Fake news often aims to manipulate public opinion, deceive readers, or generate sensationalism for personal or political gains.
Read more about mitsuri.net
The Impact of Fake News
The prevalence of fake news can have severe consequences on individuals and society. Misinformation can influence public opinion, shape political discourse, and even incite social unrest. It undermines trust in media sources and can lead to misguided decisions by individuals who believe false information to be true. Therefore, developing effective methods to detect and combat fake news is crucial in maintaining an informed and responsible society.
Fake News Detection Techniques
Machine Learning in Fake News Detection
Machine learning algorithms offer great potential in identifying patterns and features within textual data that can help distinguish between real and fake news. By analyzing various linguistic, semantic, and contextual cues, these algorithms can learn to differentiate between genuine news articles and fabricated content. Here are some common machine learning techniques used in fake news detection:
- Natural Language Processing (NLP): NLP techniques enable machines to understand and analyze human language. It involves tasks such as text classification, sentiment analysis, and topic modeling, which can be utilized to identify suspicious patterns in news articles.
- Feature Extraction: Machine learning models extract meaningful features from textual data to identify patterns unique to fake news. These features can include the frequency of certain words, grammar patterns, and stylistic differences between genuine and fake articles.
- Supervised Learning: Supervised learning algorithms are trained on labeled datasets, where each news article is classified as real or fake. The models learn from these labeled examples to make predictions on new, unlabeled data.
- Unsupervised Learning: Unsupervised learning techniques involve clustering and anomaly detection to identify patterns in the data without pre-labeled information. These methods can be useful when dealing with emerging types of fake news that may not have been seen before.
Read more about yandexgames.co
Challenges in Fake News Detection
While machine learning techniques offer promising solutions, there are several challenges in accurately detecting fake news:
- Data Quality: Obtaining reliable and diverse labeled datasets for training machine learning models can be challenging. The quality of the data used for training significantly affects the model’s performance.
- Adversarial Attacks: Those spreading fake news are constantly evolving their tactics to deceive detection algorithms. Adversarial attacks involve manipulating the content in a way that fools the machine learning models.
- Contextual Understanding: Fake news can often rely on subtle cues, including biased language, misleading headlines, or out-of-context quotes. Capturing and understanding these nuances can be challenging for machine learning models.
- Misinformation Spread: Fake news can quickly spread across various platforms and networks. Detecting and mitigating the impact of fake news in real-time is an ongoing challenge.
Conclusion
As the spread of fake news continues to be a concern, the utilization of machine learning algorithms holds immense potential in combating this issue. By leveraging techniques such as natural language processing, feature extraction, and supervised learning, we can develop robust models capable of accurately detecting fake news. However, it is crucial to address the challenges in data quality, adversarial attacks, and contextual understanding to improve the effectiveness of these models. With continued research and innovation, we can build a safer and more informed digital environment.
Read more about: ze-turf